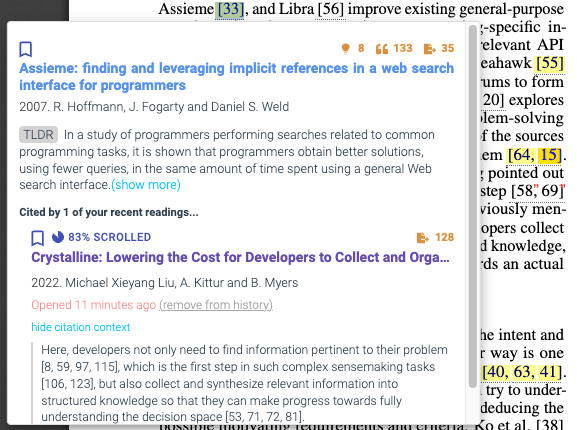
BEST PAPER AWARD
CiteSee provides a personalized paper reading experience by visually augmenting inline citations based on their connections to the current user based on their reading history, paper library, and publication records. During literature review sessions, CiteSee allows users to prioritize their exploration to focus first on inline citations most relevant to their recent readings. It also highlights inline citations to familiar papers, allowing users to keep track of their exploration and better contexturalize the current paper. Lab and field studies showed CiteSee can improve better paper discovery via inline citations, and allowed participants to have better situational awareness when conducting real-world literature reviews.